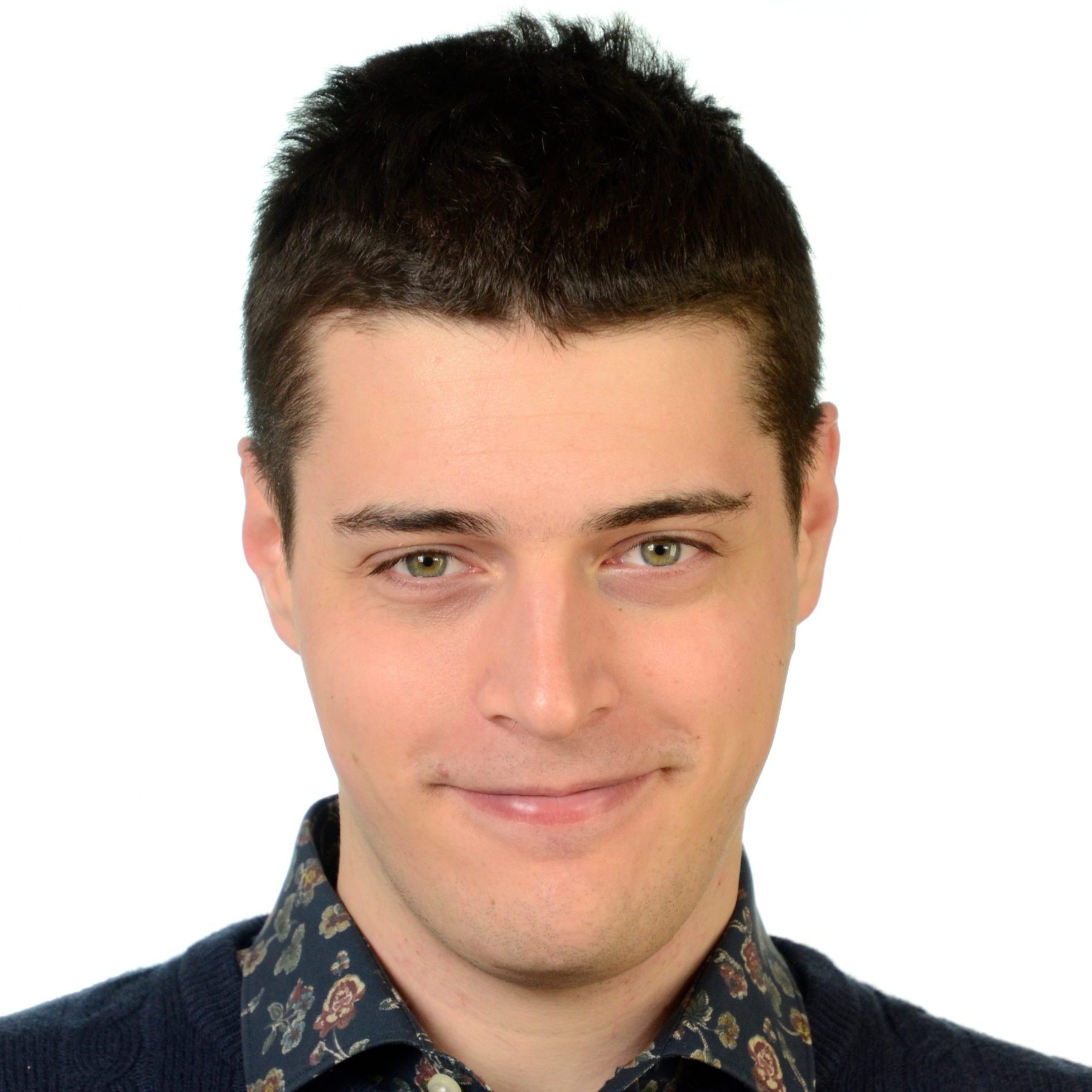
Tommaso Carraro
PhD Student
- Curriculum vitae: Download
- E-mail: tcarraro@fbk.eu
- Google Scholar: My citations
- Semantic Scholar: Profile
- DBLP: Profile
- Skype: @bmxitalia
- LinkedIn: Profile
Short bio
It is from the beginning of the high school that I love computer science and new technologies. During the years, I always loved to manage my studies and solve new problems quickly. I am determined in what I do and I constantly engaged in my tasks during the years, placing the education at the first place. Thanks to the master’s degree in computer science I discovered my passion for machine learning and research in general. For this reason, I decided to create a study plan focused on machine learning, data mining, and computer vision. University gave me the possibility to meet talented professors and researchers, even during my Erasmus exchange in Helsinki. Thanks to these people, I began to love reading and studying scientific papers about new artificial intelligence topics, in particular, I am fascinated by neural networks and their application in the medical field. During the writing of my master’s degree thesis, I worked a lot on the application of generative methods to recommender systems, creating strong foundations on Variational Autoencoders. During this period, I have also submitted three scientific papers about recommendation systems and presented one of them at the UMAP 2020 conference. At the moment, I am working as a research assistant at the Data and Knowledge Management (DKM) unit of the Fondazione Bruno Kessler (FBK) in Trento, under the supervision of Prof. Luciano Serafini. My research is focused on the application of Neural-Symbolic approaches to recommender systems. In particular, I am studying the integration of logical reasoning and deep neural networks to improve the recommendation performance and explainability. In the future, I would apply to a Ph.D. program to continue carrying on my passion and expand my knowledge about artificial intelligence. One day, I hope to become a machine learning researcher, having the opportunity to work with experienced researchers and to provide new solutions to the world.
Research topics
Neural-Symbolic Integration
Recommender Systems
Explainability of Recommender Systems